Tutorial 05: Collision Checks With Dynamic Obstacles¶
This tutorial shows you how to use the drivability checker library in order to quickly check if multiple ego-vehicle trajectories collide with a set of dynamic obstacles. The future motions of the dynamic obstacles can either be described by their predicted trajectories (trajectory prediction) or their drivable areas (set-based prediction).
1. Necessary imports and helper functions¶
We begin by importing all necessary modules and setting up helper functions.
[1]:
%matplotlib inline
import numpy as np
import matplotlib.pyplot as plt
from time import time
# commonroad
from commonroad.prediction.prediction import TrajectoryPrediction, SetBasedPrediction
from commonroad.visualization.mp_renderer import MPRenderer
#commonroad-io
from commonroad.common.file_reader import CommonRoadFileReader
# commonroad_dc
import commonroad_dc.pycrcc as pycrcc
from commonroad_dc.collision.collision_detection.pycrcc_collision_dispatch import create_collision_checker, create_collision_object
from commonroad_dc.boundary import boundary
from commonroad_dc.collision.trajectory_queries import trajectory_queries
from commonroad_dc.pycrcc.Util import trajectory_enclosure_polygons_static
def open_scenario(scenario_filename):
crfr = CommonRoadFileReader(
scenario_filename)
scenario, planning_problem_set = crfr.open()
return scenario, planning_problem_set
directory = './'
2. Collision checks¶
We demonstrate the collision checking for two CommonRoad scenarios: * USA_US101-3_3_T-1 contains the predicted trajectories of all dynamic obstacles * USA_US101-3_3_S-1 uses set-based prediction and contains the predicted drivable areas
We open and visualize the first scenario (trajectory prediction).
[2]:
# scenario names for both considered scenarios
scenario_name_trajectory_prediction="USA_US101-3_3_T-1"
scenario_name_set_prediction="USA_US101-3_3_S-1"
# open the example scenario with trajectory predictions
scenario, planning_problem_set = open_scenario(directory+scenario_name_trajectory_prediction+'.xml')
# plot the scenario
rnd = MPRenderer(figsize=(25, 10))
scenario.draw(rnd)
planning_problem_set.draw(rnd)
rnd.render()
[2]:
[<matplotlib.collections.PathCollection at 0x7f8f379ffa10>,
<matplotlib.collections.PathCollection at 0x7f8f379e6390>,
<matplotlib.collections.PathCollection at 0x7f8f379e6610>,
<matplotlib.collections.PathCollection at 0x7f8f379e6790>,
<matplotlib.collections.PolyCollection at 0x7f8f379e6890>,
Text(1.0, 0.0, ''),
<matplotlib.collections.EllipseCollection at 0x7f8f379ff9d0>,
<matplotlib.collections.EllipseCollection at 0x7f8f379e6f90>,
<matplotlib.collections.EllipseCollection at 0x7f8f37997e10>,
<matplotlib.collections.EllipseCollection at 0x7f8f37997bd0>,
<matplotlib.collections.EllipseCollection at 0x7f8f37997810>,
<matplotlib.collections.EllipseCollection at 0x7f8f379976d0>,
<matplotlib.collections.EllipseCollection at 0x7f8f379975d0>,
<matplotlib.collections.EllipseCollection at 0x7f8f37997110>,
<matplotlib.collections.EllipseCollection at 0x7f8f37997290>,
<matplotlib.collections.EllipseCollection at 0x7f8f37989e10>,
<matplotlib.collections.EllipseCollection at 0x7f8f37989bd0>,
<matplotlib.collections.EllipseCollection at 0x7f8f37989990>,
<matplotlib.collections.PatchCollection at 0x7f8f3892b410>]
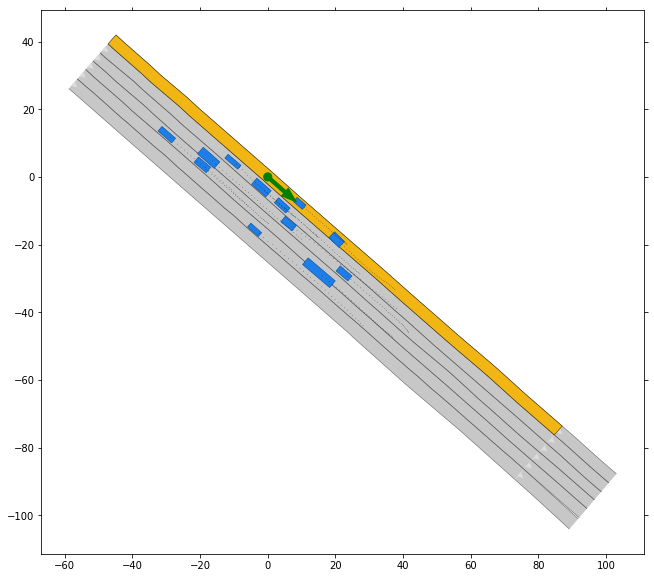
For this tutorial we have stored the waypoints for a set of 1000 trajectories in a file (USA_US101-3_3_T-1_waypoints.npz). The function get_trajectory_list()
returns the trajectory batch as a list, where each trajectory is represented as a Time-Variant Collision Object.
[3]:
def get_trajectory_list(loaded, obb_hull_preprocess=True):
traj_matrix=loaded["trajectories"].reshape(1000,-1)
start_time=np.asfortranarray(loaded["start_time_step"].astype(np.int))
half_car_length, half_car_width=loaded["car_shape"]/2
# create a trajectory batch
trajectories_batch=pycrcc.OBBTrajectoryBatch(traj_matrix,start_time, half_car_length, half_car_width)
# preprocess the trajectory batch for continuous collision detection using OBB Hull method (see also Tutorial 03)
if obb_hull_preprocess:
trajectories_batch.preprocess_()
return trajectories_batch.to_tvobstacle()
We load the data from the *.npz
file and generate the trajectory batch as a list.
[4]:
data=np.load(scenario_name_trajectory_prediction+"_waypoints.npz")
loaded_data = {"car_shape": data["car_shape"], "trajectories": data["trajectories"], "start_time_step": data["start_time_step"]}
data.close()
trajectories = get_trajectory_list(loaded_data)
waypoints=loaded_data["trajectories"]
car_shape=loaded_data["car_shape"]
car_half_length, car_half_width = (car_shape/2)
After loading the trajectory batch, we create the road boundary (see Tutorial 03: road compliance checking for details). The scenario and an exemplary part of the trajectory batch is visualized below.
[5]:
# create the road boundary with default method uses oriented rectangles
road_boundary_obstacle, road_boundary_sg_rectangles=boundary.create_road_boundary_obstacle(scenario)
road_polygons = boundary.create_road_polygons(scenario, method='whole_polygon', triangulate=False)
# Draw an exemplary part of trajectory batch (here: 50 trajectories) and the road boundary
n_traj_draw=50
offset=350
rnd = MPRenderer(figsize=(25, 10))
scenario.draw(rnd)
road_boundary_sg_rectangles.draw(rnd)
for tvo in trajectories[offset:offset+n_traj_draw]:
tvo.draw(rnd, draw_params={'facecolor': 'green'})
rnd.render()
[5]:
[<matplotlib.collections.PathCollection at 0x7f8f378b3650>,
<matplotlib.collections.PathCollection at 0x7f8f378b3fd0>,
<matplotlib.collections.PathCollection at 0x7f8f379e6510>,
<matplotlib.collections.PathCollection at 0x7f8f378a73d0>,
<matplotlib.collections.PolyCollection at 0x7f8f378a7550>,
<matplotlib.collections.EllipseCollection at 0x7f8f378a7bd0>,
<matplotlib.collections.EllipseCollection at 0x7f8f378a7e10>,
<matplotlib.collections.EllipseCollection at 0x7f8f3781d090>,
<matplotlib.collections.EllipseCollection at 0x7f8f3781d2d0>,
<matplotlib.collections.EllipseCollection at 0x7f8f3781d550>,
<matplotlib.collections.EllipseCollection at 0x7f8f3781d7d0>,
<matplotlib.collections.EllipseCollection at 0x7f8f3781da10>,
<matplotlib.collections.EllipseCollection at 0x7f8f3781dc50>,
<matplotlib.collections.EllipseCollection at 0x7f8f3781dc10>,
<matplotlib.collections.EllipseCollection at 0x7f8f37810090>,
<matplotlib.collections.EllipseCollection at 0x7f8f378102d0>,
<matplotlib.collections.EllipseCollection at 0x7f8f37810510>,
<matplotlib.collections.PatchCollection at 0x7f8f3783b910>]
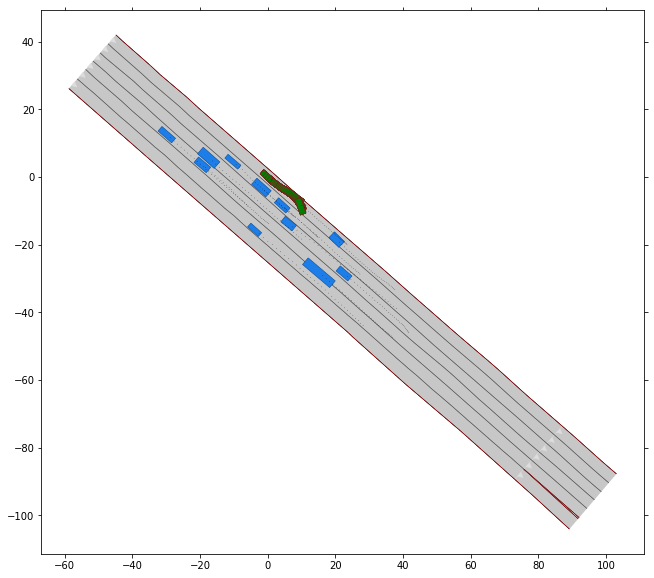
2.1. Check if trajectory is within the road (static collision checking)¶
We can check the trajectory batch for road-compliance by performing a static collision check with the road boundary, as demonstrated in Tutorial 03: road compliance checking.
Simplified approach (check for the collision of each trajectory with the road boundary)¶
[6]:
# check computation time over ten runs
num_trials=10
cur_time_1=time()
for i in range(num_trials):
# check if the trajectory collides with the road boundary
ret=trajectory_queries.trajectories_collision_static_obstacles(trajectories, road_boundary_sg_rectangles, method='grid', num_cells=32, auto_orientation=True, optimize_triangles=True)
cur_time_2 = time()
print("%s out of %s checked trajectories do not collide with the road boundary." % (ret.count(-1), len(trajectories)))
print("Time for %s trajectory checks: " % (len(trajectories)) + str((cur_time_2-cur_time_1)/num_trials)+ " sec.")
620 out of 1000 checked trajectories do not collide with the road boundary.
Time for 1000 trajectory checks: 0.005657196044921875 sec.
Full approach (check if the vehicle is within the road at the first time step, then check if the trajectory collides with the road boundary)¶
[7]:
# check computation time over ten runs
num_trials=10
cur_time_1=time()
for i in range(num_trials):
candidate_trajectories=list()
#select only the trajectories for which the vehicle is within the road at the first timestep
candidate_trajectories=trajectory_queries.filter_trajectories_polygon_enclosure_first_timestep(trajectories,road_polygons)
# check if the trajectory collides with the road boundary
ret=trajectory_queries.trajectories_collision_static_obstacles(candidate_trajectories, road_boundary_sg_rectangles, method='grid', num_cells=32, auto_orientation=True, optimize_triangles=True)
cur_time_2 = time()
print("%s out of %s checked trajectories are fully within the road." % (ret.count(-1), len(trajectories)))
print("Time for %s trajectory checks: " % (len(trajectories)) + str((cur_time_2-cur_time_1)/num_trials)+ " sec.")
620 out of 1000 checked trajectories are fully within the road.
Time for 1000 trajectory checks: 0.014472603797912598 sec.
2.2. Dynamic collision checking¶
Afterwards, we can check whether the trajectories collide with the dynamic obstacles in the scenario using either trajectory predictions (Section 2.2.1.) or set-based predictions (Section 2.2.2)
The function get_scenario_dynamic_obstacles_list()
returns the dynamic obstacles in the scenario as a list of Time-Variant Collision Objects. We preprocess the trajectories of other vehicles for continuous collision checking using trajectory_preprocess_obb_sum()
, which creates a conves hull around the occupancies of two subsequent time steps.
[8]:
def get_scenario_dynamic_obstacles_list(scenario):
dyn_obstacles_list=list()
for dyn_obst in scenario.dynamic_obstacles:
if isinstance(dyn_obst.prediction,TrajectoryPrediction):
co=create_collision_object(dyn_obst.prediction)
# preprocess using obb hull for continuous collision detection
co, err=trajectory_queries.trajectory_preprocess_obb_sum(co)
dyn_obstacles_list.append(co)
else:
if isinstance(dyn_obst.prediction,SetBasedPrediction):
co=create_collision_object(dyn_obst.prediction)
dyn_obstacles_list.append(co)
else:
raise Exception('Unknown dynamic obstacle prediction type: ' + str(type(dyn_obst.prediction)))
return dyn_obstacles_list
We get all dynamic obstacles in the scenario with the trajectory prediction:
[9]:
dynamic_obstacles=get_scenario_dynamic_obstacles_list(scenario)
Then, we can check the trajectory batch for collision with other dynamic obstacles.
[10]:
# check computation time over ten runs
num_trials=10
cur_time_1=time()
for i in range(num_trials):
res_dynamic = trajectory_queries.trajectories_collision_dynamic_obstacles(trajectories, dynamic_obstacles, method='box2d')
cur_time_2 = time()
print("%s out of %s trajectories do not collide with the other vehicles" % (res_dynamic.count(-1), len(trajectories)))
print("Time for %s trajectory checks: " % (len(trajectories)) + str((cur_time_2-cur_time_1)/num_trials)+ " sec.")
820 out of 1000 trajectories do not collide with the other vehicles
Time for 1000 trajectory checks: 0.00523533821105957 sec.
We open the second scenario which uses set-based predictions for the dynamic obstacles.
[11]:
# open the example scenario with set-based predictions
scenario, planning_problem_set = open_scenario(directory+scenario_name_set_prediction+'.xml')
Again we create the road boundary and visualize the scenario.
[12]:
# create road boundary with default method using oriented rectangles
road_boundary_obstacle, road_boundary_sg_rectangles=boundary.create_road_boundary_obstacle(scenario)
road_polygons = boundary.create_road_polygons(scenario, method='whole_polygon', triangulate=False)
# Draw an exemplary part of trajectory batch (here: 50 trajectories) and the road boundary
n_traj_draw=50
offset=350
rnd = MPRenderer(figsize=(25, 10))
scenario.draw(rnd)
road_boundary_sg_rectangles.draw(rnd)
for tvo in trajectories[offset:offset+n_traj_draw]:
tvo.draw(rnd, draw_params={'facecolor': 'green'})
rnd.render()
[12]:
[<matplotlib.collections.PathCollection at 0x7f8f36d91e90>,
<matplotlib.collections.PathCollection at 0x7f8f376fd490>,
<matplotlib.collections.PathCollection at 0x7f8f36de4050>,
<matplotlib.collections.PathCollection at 0x7f8f36de4150>,
<matplotlib.collections.PolyCollection at 0x7f8f36de40d0>,
<matplotlib.collections.PatchCollection at 0x7f8f36cbf590>]
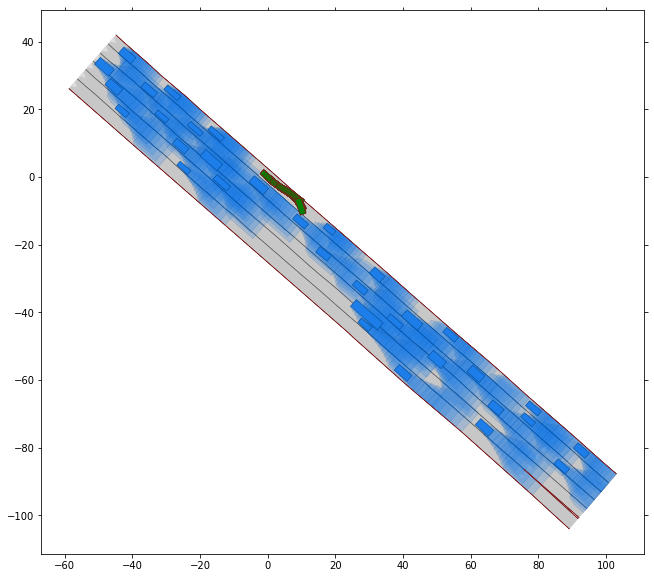
We retrieve all dynamic obstacles in the scenario.
[13]:
dynamic_obstacles=get_scenario_dynamic_obstacles_list(scenario)
Finally, we can check the trajectory batch for collision with the set-based predictions of the other traffic participants.
[14]:
# check computation time over ten runs
num_trials=10
cur_time_1=time()
for i in range(num_trials):
res_dynamic = trajectory_queries.trajectories_collision_dynamic_obstacles(trajectories, dynamic_obstacles, method='grid')
cur_time_2 = time()
print("%s out of %s trajectories do not collide with the set-based predictions" % (res_dynamic.count(-1), len(trajectories)))
print("Time for 1000 trajectory checks: " + str((cur_time_2-cur_time_1)/num_trials)+ " sec.")
1000 out of 1000 trajectories do not collide with the set-based predictions
Time for 1000 trajectory checks: 0.0070689678192138675 sec.